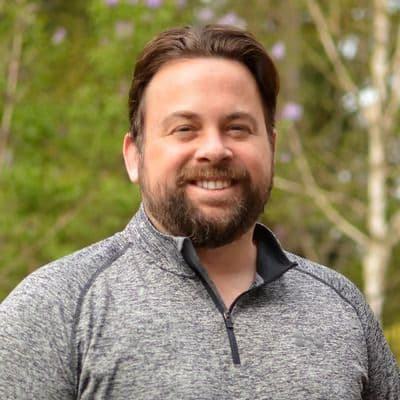
Crafty Hacks with Convex MCP
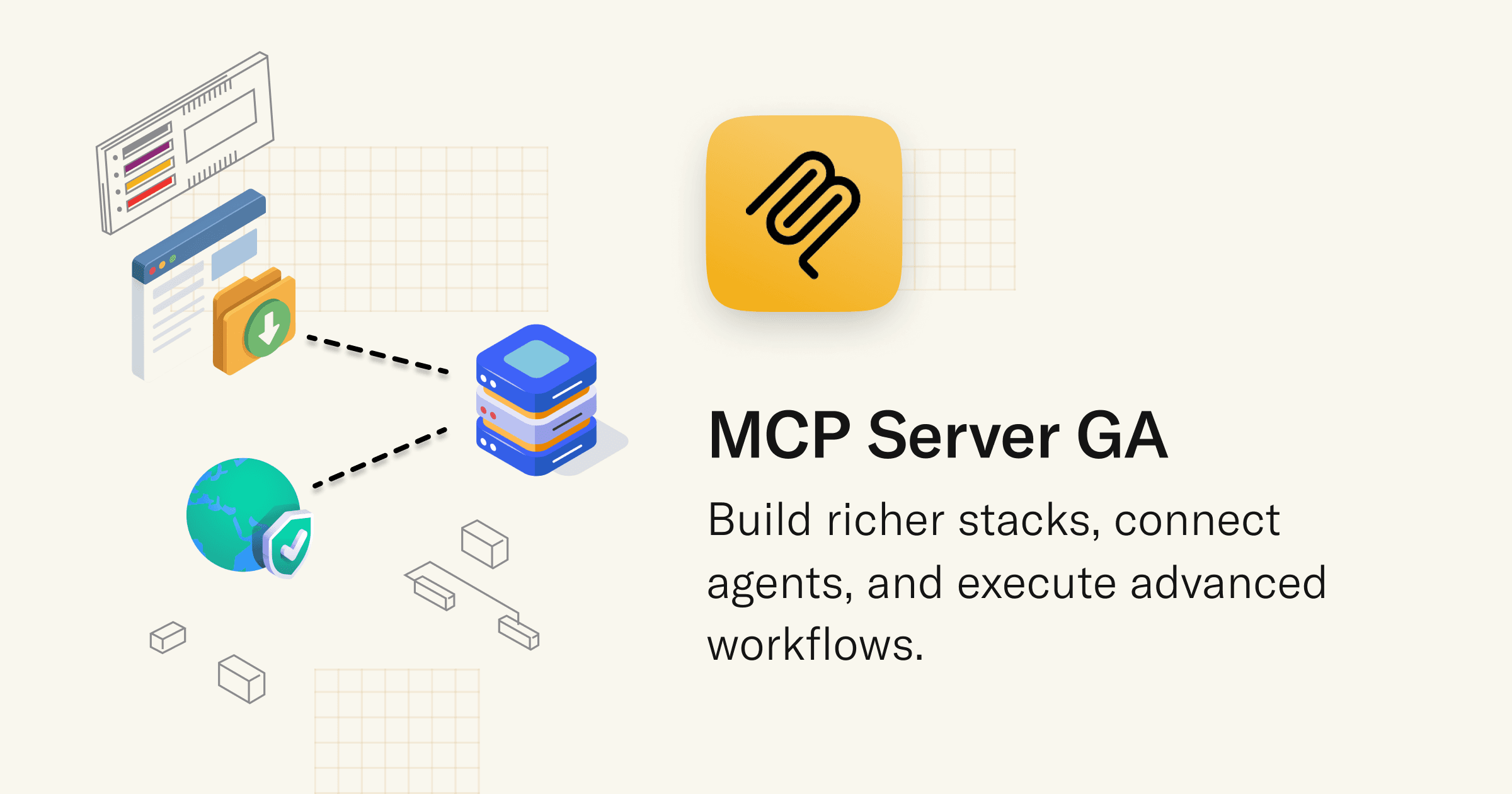
MCP (Model Context Protocol) has been making waves recently by enabling AI agents to interoperate closely with all manner of services and data.
As chance would have it, Convex is a service with data... your app’s data! So MCP provides an excellent way to allow LLMs to work even more effectively with your Convex backend.
Therefore, we’re thrilled to announce general availability of the Convex MCP server.
Our MCP server exposes numerous useful tools to agents, such as:
- tables: Lists your project’s database tables and declared and/or inferred schema.
- data: Paginates over all the data in a given table.
- functionSpec: Enumerates all your backend functions, including arguments and return values.
- run: Invokes one of your backend functions.
- runOneoffQuery: Executes a new, ephemeral query the agent writes in a read-only context to go deeper into your backend data.
Now, LLM-powered agents are clever critters. So with the right prompting, they can compose these tools to execute surprisingly complex jobs.
To give you a taste of what’s possible, let’s dive deep into two MCP-powered scenarios using Cursor’s agent mode.
Make sure you have Convex's latest Cursor rules installed in your project
for the best agent performance. (If you created your project with a recent version of npx create convex
, they were installed automatically.)
Crafty Hack #1: Magical Migrations
Migrating databases is error prone, frustrating work. And if you want to do a zero downtime, online migration, it’s even more tedious.
Consider the steps for adding a single new field to an existing table:
- Add the field as optional. All the records in the database do not have this field yet, so it can’t be required!
- Update your application code to start populating this new field when future records are added or changed.
- Deploy your project. So you at least know all future writes have the field set.
- Define and kick off a backfill job to traverse all historical records and set the field.
- Finally, mark the field as required and do one more deployment.
After these careful steps, your new field exists, is set appropriately, and your code is clean, as though nothing ever changed.
What a pain. Let’s teach the agent to do this whole thing for us instead.
First, in Cursor, head to Cursor Settings > General > Project Rules
and add the following MDC file to your project. It teaches the agent how to do this migration workflow on Convex:
Now that we’ve given the Cursor agent the appropriate guidelines, let’s have it go to work for us. Here’s a migration adding a geospatial positions
field to existing database records in a table of geographical locations:
While automating linear workflows is valuable, MCP's true power comes from tapping into AI's capacity for reasoning...
Crafty Hack #2: AI vs. AI
It’s wonderful to have AI run our migrations for us. But admittedly, we merely taught it how to automate a sequence of steps that a simple script could solve.
Now let’s do something more audacious: let’s ask the agent to make a subjective judgement on the accuracy of an AI-driven classification system.
Assume we have a basic contact management app that collects information about new friends you meet: their name, email, and a short bio.
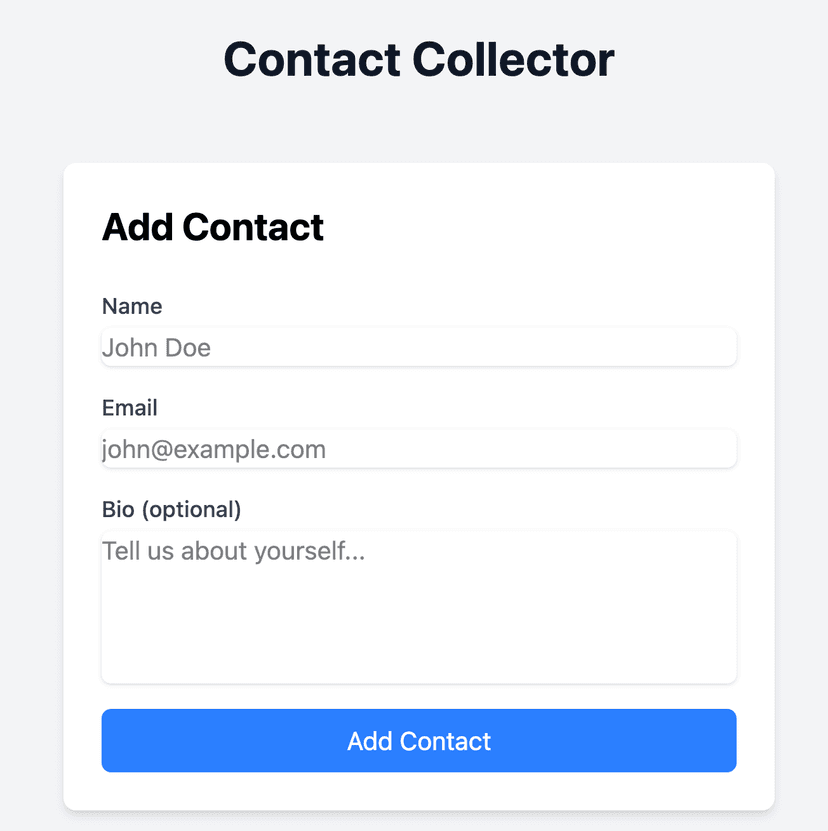
We’ll store these contact attributes in a Convex table of course—but then we’ll also have a “category” field for placing this person into one of three personality groups: sporty, bookish, or social.
Here’s the schema:
1import { defineSchema, defineTable } from "convex/server";
2import { v } from "convex/values";
3
4export default defineSchema({
5 contacts: defineTable({
6 name: v.string(),
7 email: v.string(),
8 bio: v.optional(v.string()),
9 category: v.optional(
10 v.union(v.literal("sporty"), v.literal("bookish"), v.literal("social")),
11 ),
12 }).index("by_email", ["email"]),
13});
14
When the new contact form is submitted, our app uses a mutation called api.contacts.add
to do two things:
- Insert the contact into the database
- Kick off a background action that asks GPT to categorize this contact based on the provided bio.
Here’s the relevant section from the add
mutation:
1 // Insert the contact into the database.
2 const id = await ctx.db.insert("contacts", {
3 name: args.name,
4 email: args.email,
5 bio: args.bio ?? "",
6 });
7
8 // Schedule categorization if the bio is provided.
9 if (args.bio) {
10 await ctx.scheduler.runAfter(0, internal.categorize.categorizeContact, { contactId: id });
11 }
12
After getting the categorization response back from OpenAI, this background categorizeContact
action uses a mutation to write the chosen category back out to the database.
Now that we have this wired up, let’s ask Cursor’s agent to try this out and make sure everything seems reasonable:
Imagine that: using AI’s capacity for judgement to get feedback on the quality of an AI-driven, nondeterministic process!
Get started with Convex MCP
These two scenarios just scratch the surface of what’s possible when you have agents interact with your Convex projects over MCP.
So set up your MCP server and get building! If you figure out more cool workflows, come join our community and share!
Convex is the backend platform with everything you need to build your full-stack AI project. Cloud functions, a database, file storage, scheduling, workflow, vector search, and realtime updates fit together seamlessly.